Image: Overview of human influenza response module expression patterns. The red-blue heat map shows mean expression of all genes in each module. The more red (blue) an entry, the higher (lower) expression in infected vs. mock-treated cells. See full figure.
Understanding how the human immune system responds to different types of pathogenic infections is key to improving treatment strategies in infectious diseases. An important component of mounting pathogen-specific immune responses is the transcriptional regulatory network that controls what genes must be activated under specific infections to the host cell. However, our understanding of this regulatory network and how it drives context-specific transcriptional programs is incomplete.
Advances in functional genomics techniques are rapidly expanding our repertoire of omic datasets that measure different components of these networks. A major challenge is to systematically combine these different types of datasets to identify the regulatory networks driving host response than can then be used to improve our understanding of the immune system.
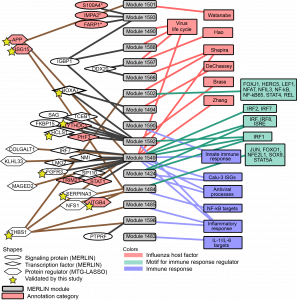
To address this gap, Systems Biology researchers at WID (Sushmita Roy and Deborah Chasman) and the Influenza Research Institute (Yoshihiro Kawaoka, Amie J. Eisfeld, Kevin B. Walters, and Tiago J.S. Lopez), used machine learning approaches to integrate high-throughput mRNA- and protein measurements and protein-protein interaction networks to identify virus and pathogenicity-specific regulatory networks. They inferred regulatory networks and gene expression modules (groups of genes that are co-expressed across different pathogenic virus strains) for human cell line and mouse host systems, which recapitulated several known regulators and pathways of the immune response and viral life cycle. They used the networks to prioritize important regulators of host response as well as to study time point and strain-specific subnetworks. The analysis predicted several novel regulators, both at the mRNA and protein levels, a number which were tested experimentally and shown to significantly impact viral replication.
As systems biology studies expand to more infectious agents and host systems, approaches such as this are going to be increasingly useful to assemble a comprehensive picture of the mechanisms responsible for healthy and disease states, and ultimately guide the design of effective therapeutics.
The full paper, titled Integrating Transcriptomic and Proteomic Data Using Predictive Regulatory Network Models of Host Response to Pathogens is published in the July 2016 issue of PLOS Computational Biology.
— Deborah Chasman
You must be logged in to post a comment.