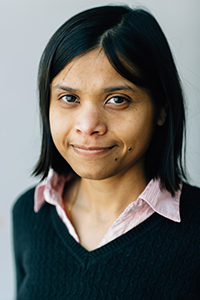
Computational methods to model cellular networks
Education
- Ph.D. Computer Science, University of New Mexico
Research Description
Sushmita Roy's research focuses on developing statistical computational methods to identify the networks driving cellular functions by integrating different types of genome-wide datasets that measure different aspects of the cellular state.
Roy is interested in identifying networks under different environmental, developmental and evolutionary contexts, comparing these networks across contexts, and constructing predictive models from these networks.
Specifically, some research topics of interest are:
- Inference of structure and function of regulatory networks
- Comparative analysis of expression modules across species
- Evolution of gene regulation
- Relational learning to predict function
- Modeling condition-specific functional behavior
- Learning causal networks
- Predictive models of phenotypic response