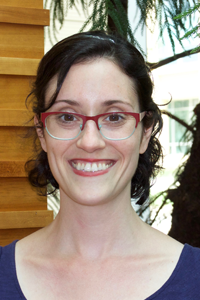
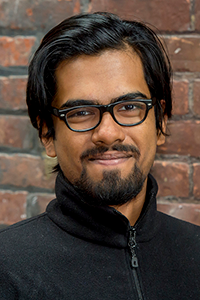
While looking at a graph about fruit, it may seem intuitive to associate a bar of blue to blueberries and yellow to bananas, but are there connections between color and abstract concepts such as driving, comfort, efficiency, or reliability?
Visualizations where colors do not match expectations are hard to interpret. For example, reading a bar graph where the bananas are coded purple and the blueberries are red can be confusing, even if there is a clear legend, because the color-concept mappings do not align with people’s expectations. Previously, it was thought that these kinds of expectations only existed for “colorable” concepts – concepts that have highly specific associations.
However, Karen Schloss’s group at the University of Wisconsin-Madison’s Wisconsin Institute for Discovery has discovered that color expectations can be shaped for concepts previously considered non-colorable, like driving, comfort, efficiency, and reliability. These expectations are based on color-concept associations, which can come from personal experiences, location, and cultural backgrounds.
Associations between colors and concepts can influence how someone finds meaning from information visualizations, such as graphs, maps, diagrams, and signs. The Schloss group developed a model that specifically deconstructs how visual cues can be selected for any abstract or physical concept for the purpose of designing visualizations that people can easily interpret. Their “semantic discriminability theory” suggests that if a set of concepts has color-concept associations that are different enough, it will be possible to select colors that allow people to infer the meanings of these colors in information visualizations.
If three criteria are met, the research suggests that a color palette for a group of concepts can be defined, in order to design interpretable palettes. First, there needs to be some specificity. At least some concepts in a concept set must have some colors that are more associated with that concept than other colors, such as red being more associated than pink with the concept driving. These associations can be determined using human judgments, image statistics, and natural language databases. Secondly, there needs to be a wide range of colors available for use in the visualization to allow for enough contrast. Third, the color ranges must be large enough to incorporate distinguishable differences in particular colors. For instance, there should be enough blues in the color palette to differentiate between a night or morning sky.
In a recent study led by Kushin Mukherjee in the Schloss group, participants were shown graphs containing four colored bars and four concept labels. They were asked to guess which label matched with each colored bar. At the start of each trial, a set of four concepts, from fruits, vegetables, activities or properties were centered above the graph and the participants chose what color they thought matched each color in the graph. The researchers investigated if people could accurately interpret those colors, both for concepts previously considered colorable, such as fruits, and for concepts previously considered non-colorable, such as activities. Although color was the focus in this study, other visual features like shape, orientation or visual texture could be used.
All activities (e.g., sleeping, driving, leisure) had at least one instance that was as accurate as the fruits, even though those activities were previously considered non-colorable. These results suggest that any concept has the potential to be meaningfully encoded using color, provided the color has enough meaningful difference with other colors in a palette. These findings mean that colors are more robust for visual communication than previously thought.
Mukherjee and his collaborators have published their findings in IEEE Transactions on Visualization and Computer Graphics. Understanding how people absorb meaning from visual features, and predicting the meaning they attribute to color in any context is filled with possibility. Processing information faster could help convey meaning more quickly and accurately, reduce confusion and guesswork, and promote more effective and efficient communication. For example, better weather visualizations on a television news program would help people more quickly and accurately understand and make decisions on how to prepare for the weather.
-Laura C. Red Eagle
This project was supported by the UW–Madison Office of the Vice Chancellor for Research and Graduate Education, Wisconsin Alumni Research Foundation, and the National Science Foundation (BCS-1945303).
You must be logged in to post a comment.